Data Structure Assignment代写
Keywords:Data,Structure,Assignment,代写,数
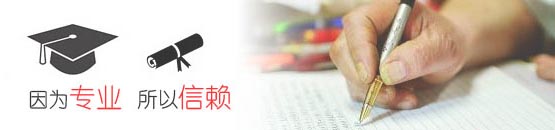
数据挖掘是从不同的角度分析数据并将其归纳为有用信息的过程。数据挖掘或知识发现,是对大量数据进行挖掘和分析,提取数据含义的计算机辅助过程。数据集的非常高的维数,如微阵列数据,对现有的数据挖掘算法的高效处理带来了巨大的挑战。在高维空间中的数据管理带来的并发症,如退化的查询处理性能,这种现象也被称为维数灾难。 降维(DR)解决了这个问题,通过方便的嵌入数据从高维到低维空间。降维的方法给出了一个最佳的解决方案,这些高维数据的分析。减少过程是减少变量计数到少数类别的行动。减少变量是新定义的变量,这是线性或非线性组合变量的组合。从不寻常的尺寸,空间,类和变量的变量减少到一个明确的维度或分类提取。 降维被认为是一个强大的方法,细化高维数据。传统的统计方法部分地关闭由于增加的观测值,主要是由于与每个观察相关联的变量的数目增加。降维的高维数据转化成一个有意义的表示(HDD)降维。主模式分析(PPA)开发了特征提取和特征分类。 基于降维多层次马氏(MMDR),这是能够降低维数的同时保持精度高,能够有效地处理大型数据集。本研究的目的是发现蛋白质折叠的同时考虑的顺序信息和三维折叠的结构信息。此外,所提出的方法减少了错误率,显着上升的吞吐量,减少丢失的项目,最后的模式分类。
Data Structure Assignment代写
Data mining is the process of analyzing data from different perspectives and summarizing it into useful information. Data mining or knowledge discovery, is the computed assisted process of digging through and analyzing enormous sets of data and then extracting the meaning of data. Data sets of very high dimensionality, such as microarray data, pose great challenges on efficient processing to most existing data mining algorithms. Data management in high dimensional spaces presents complications, such as the degradation of query processing performance, a phenomenon also known as the curse of dimensionality. Dimension Reduction (DR) tackles this problem, by conveniently embedding data from high dimensional to lower dimensional spaces. The dimensional reduction approach gives an optimal solution for the analysis of these high dimensional data. The reduction process is the action of diminishing the variable count to few categories. The reduced variables are new defined variables which are the combinations of either linear or non-linear combinations of variables. The reduction of variables to a clear dimension or categorization is extracted from the unusual dimensions, spaces, classes and variables. Dimensionality reduction is considered as a powerful approach for thinning the high dimensional data. Traditional statistical approaches partly calls off due to the increase in the number of observations mainly due to the increase in the number of variables correlated with each observation. Dimensionality reduction is the transformation of High Dimensional Data (HDD) into a meaningful representation of reduced dimensionality. Principal Pattern Analysis (PPA) is developed which encapsulates feature extraction and feature categorization. Multi-level Mahalanobis-based Dimensionality Reduction (MMDR), which is able to reduce the number of dimensions while keeping the precision high and able to effectively handle large datasets. The goal of this research is to discover the protein fold by considering both the sequential information and the 3D folding of the structural information. In addition, the proposed approach diminishes the error rate, significant rise in the throughput, reduction in missing of items and finally the patterns are classified.